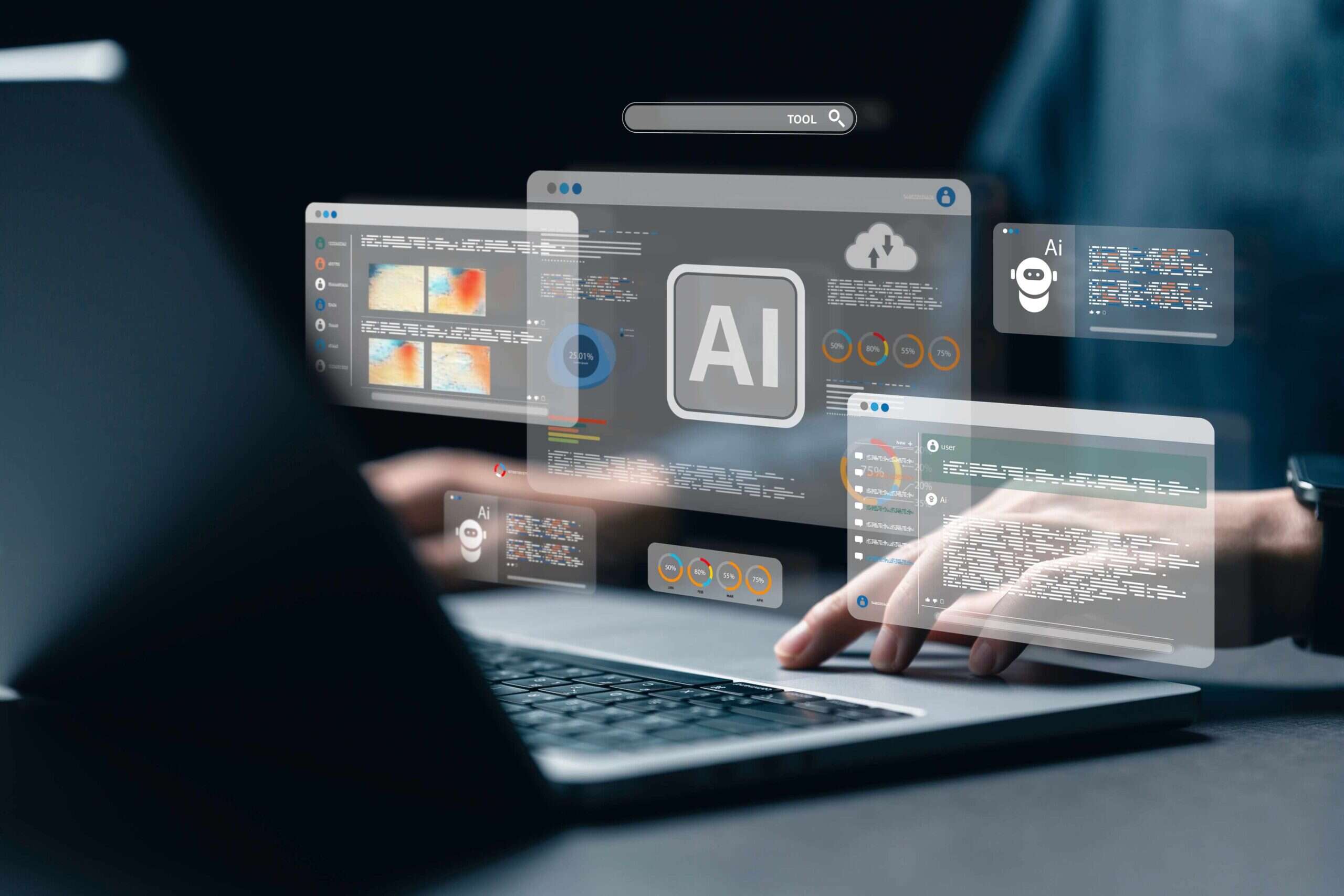
In 2022, OpenAI launched ChatGPT, its pioneering large language model (LLM) chatbot capable of generating human-like responses to prompts. The model – easily accessible and mostly free to use – took the world by storm as it marked the beginning of a new, momentous era for generative AI.
But generative AI isn’t a new affair. While its capabilities have been honed with time, its inception dates back to the early days of machine learning, when the idea of generating original content was put into words by Alan Turing’s timeless query, “Can machines think?”
Today, the advancement of deep machine learning, the development of natural language processing and the hundreds of gigabytes fed to models as training data allow generative AI models to produce text, images and other content.
These advances don’t come without risks and limitations, though. Along with the breakthroughs, recent years have witnessed waves of lawsuits directed against AI companies training their models with copyrighted data, misinformation claims and instances of defaming or discriminating output.
Not only is generative AI now practically ubiquitous, but it continues to develop in increasingly complex ways.
How does generative AI work?
The foundational component of generative AI is training data. The more data is fed to a machine, the more it will be able to draw from to generate outputs. It should thus come as no surprise that ChatGPT 4 was trained with no less than 570GB of text data from around the internet.
Daniel Leufer, a senior policy analyst at Access Now, explains to Tech Monitor that “generative AI systems are trained on huge datasets of text, images, and other media in order to produce similar but synthetic content.” He says that “these systems also make predictions about the text likely to follow a given prompt, but they generate content as their output, hence the term ‘generative AI.’”
Sophisticated generative AI models can learn from that data and respond to prompts by drawing relevant information from it – and replicating it appositely. Depending on how the AI system is built, output can be in the form of text, photos, videos, images or audio.
But output is not the only thing that has significantly evolved in recent years. The ability for AI models to receive human-like input has opened the door to an entirely new era for user experience, which went from prompting information through APIs or coding to advanced natural language processing (NLP).
NLP models powered by generative AI are now able to perform tasks such as information retrieval, sentiment analysis, information extraction and question-answering.
How is generative AI used?
There are two main forms under which generative AI can be found, LLMs and multi-modal models. LLMs, such as ChatGPT, “generate plausible-sounding text in response to a human prompt in the form of a request,” Leufer explains. On the other hand, multi-modal models can take prompts or generate output under different formats, including audio, video and images.
The expanding capabilities of generative AI have catapulted it “into the public consciousness” Leufer says. In fact, the technology is now used across sectors and for different scopes.
In 2023, a Deloitte survey found that around one in ten UK adults have used generative AI for work purposes, while a 2024 study by LexisNexis shows that 26% of legal professionals use it more than once a month in law firms.
This is because the use cases of generative AI range particularly widely, from customer service and technical support to optimisation of chip design and completion of repetitive tasks – for example. In fact, implementing such sophisticated models in different businesses and organisations has improved content production, performance optimisation and service quality.
What are the risks of generative AI?
Models “cannot actually understand the inputted or outputted data,” Leufer explains, which “can lead them to replicate harmful biases, including outright racist and sexist assumptions.” Some instances of harmful content generated by AI include discriminating claims but also misinformation. LLMs “regularly produce completely false information in response to prompts,” Leufer says.
This issue easily escalates further as existing systems often act as foundation models for other applications and services, allowing “those same biases [to] find their way into other systems and apps,” Leufer explains.
Any output is, in some way, a reproduction of the content used to train the models – which sparks an existential issue for generative AI. A lot of the data scraped on the web to train the models is copyrighted – from books to sounds and images – and is often recognisable in the content generated by AI, without credits. Several copyright lawsuits filed by publishers and artists against AI companies in recent months show the extent to which generative AI is a potential threat to the creative industries.
But between legal limitations and ethical concerns, generative AI keeps evolving fast. And while Alan Turing’s query might not be the order of the day, it is certainly becoming more relevant – in more ways than one.