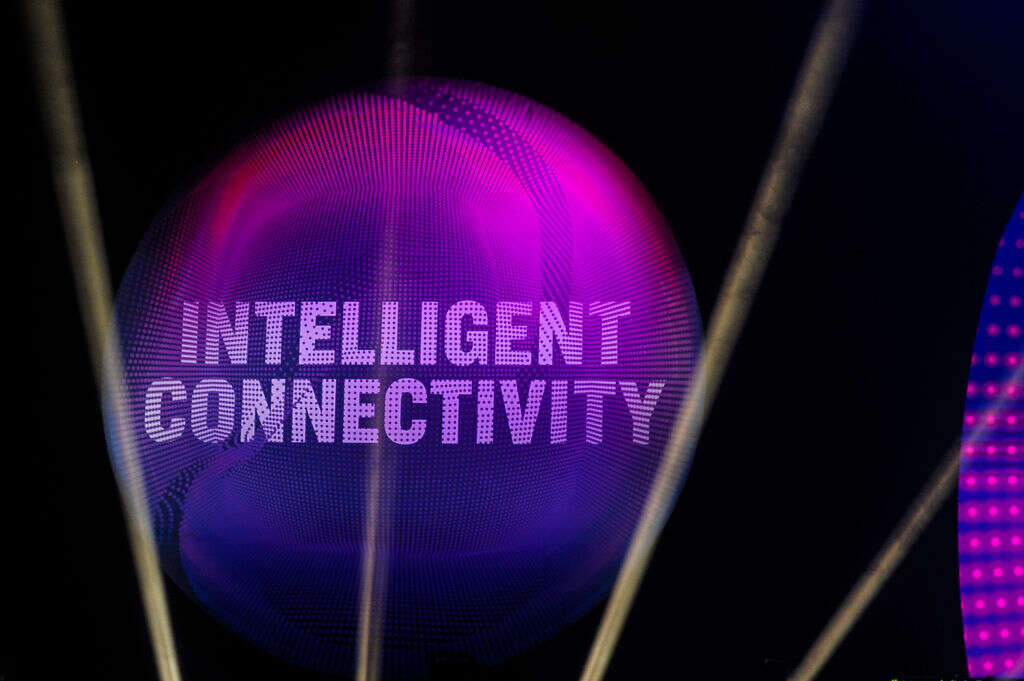
Artificial Intelligence is crucial to secure edge computing networks. The number of sensors and other devices at the edges of networks is already in the billions and is growing at pace. Each device is a potential entry point for a cyber criminal to gain malicious access to an organisations’s system. Active monitoring of such edge devices by AI and Machine Learning is the most reliable way of maintaining appropriate levels of security throughout a corporate system. But what sorts of problems does the use of the AI itself bring to an organisation?

What is secure edge computing?
Edge computing in its current form means devices at the end of a network like sensors, phones or laptops, of which there are already billions. As the use of 5G broadband becomes more widespread, the capacity to connect these billions of devices and to process the data they create will grow. This data will be too much to process efficiently in the cloud and will be processed at the edge instead.
This brings both advantages and disadvantages. The amount of lag, or latency time, will be dramatically lessened if data can be stored closer to those interacting with it, as it won’t need to be transported to cloud storage and back again to complete a transaction. In some cases this is crucial. For example, on an oil rig companies cannot afford to wait for data coming from sensors to be routed back to the cloud for analysis, explains analyst at Gartner Donald Feinberg at a recent Data and Analytics Summit. “I don’t want to have to send data back to the home office to get the result and then send back an alert that says ‘uh oh, you’ve got a real problem'” he said. “By then the oil rig already blew up, it’s that simple.”
This is also a traffic issue, explains Bola Rotibi, research director at industry analyst firm CCS Insight. “The whole point about processing data at the edge is to ensure that you don’t have too much noise going across the network. So in fact, you’re only sending things back that need to be sent back.”
Cybersecurity risks at the edge
However, storing data at numerous edge devices brings with it a tranche of new cybersecurity issues that must be considered before edge computing is fully implemented. Each device on a distributed network is a potential entry point for a cyber criminal and these dangers are already very real. Forty-two percent of executives surveyed by CapGemini reported that cybersecurity incidents through IoT devices had increased on average by 16%. “If you think about how many devices are being connected today, it’s millions,” explains course director of AI for cybersecurity at Oxford University and founder of cyber security platform CyberPulse Raj Sharmer. “What happens if one of the devices is compromised? That’s a big loss for the company. If one device is compromised the attacker can use it to get into the network and potentially infect the other billion devices on there,” he says.
Issues with data storage and backup are not uncommon in edge computing either. Localised devices often have limited storage options and may be unable to back up critical files. In this case only the crucial processing data is stored at the edge, which makes its security all the more important. In retail, for example, edge computing networks require automated data leakage monitoring and insight into data storage vulnerabilities to stem exploitation of Personally Identifiable Information (PII), reports AT&T.
Edge security concerns do not only live in the virtual world. “When we think about the edge, it is not just the computing at the edge, it is the physical security,” says Rotibi. Data can be stolen or tampered with or the device could be broken. It could be possible to steal an entire database simply by removing the memory stick from the edge computing source. Devices could be tampered with to introduce malware through such physical access also, warns security company Atos in a report.
How AI can mitigate these issues
Countermeasures range from secure data center processes like access controls and audio/video monitoring, to monitoring temperature changes and movement or protecting against natural disasters like fires and floods, states the Atos report.
Misbehaviour detection solutions are another way to monitor large amounts of smart devices within a network. An algorithm can monitor a smart network and learn to detect odd behaviour. “AI works on the patterns of data,” explains Sharma. “Solutions on the edge can be deployed to detect the behaviour of the threat actors. There are so many devices that we need to make sure the AI can detect the behaviour and send an alert back to the system.” This has been called User Entity Behaviour Analytics by AT&T. “These are tools that augment or supplement what the security practitioner is doing, creating faster detection of anomalies, leaving that practitioner to focus on other higher level work,” clarifies Tawnya Lancaster, lead in security trends research at AT&T.
Decentralising data storage can weaken password control as individual employees may choose passwords that are easy to crack or opt to bypass multi factor authentication, mentions Sharmer. “We have so many passwords to remember, I think we need to find another way, probably biometric authentication, rather than remembering the password,” he agrees. One good way of mitigating this risk with AI is to carry out what Sharma calls ‘compliance checks’. “If there is something the user isn’t doing, we can put some mechanism on the device that will alert back to the system to say that the person, or device, is not compliant.”
Once devices are optimised with artificial intelligence, however, they become more dangerous as they can be weaponised by a malicious actor, counters Rotibi. “With adding intelligence to devices you’re heightening their level of processing, which means they can actually do stuff, which makes them an attack surface.” The more devices interconnect and transmit data, the more dangerous they can potentially be. “With more processing capability comes more opportunity for an actor to gain control,” she says.
Ultimately AI is a useful tool to help tackle the “big data challenge” as Lancaster calls it. However, she explains, “it is not a silver bullet. Remember, machine learning is only as good as the algorithms that are being trained by the practitioners and the data that is being fed into those algorithms.
“If the data that is being collected from an organisations environment isn’t high quality, the ML output will not be high quality.”