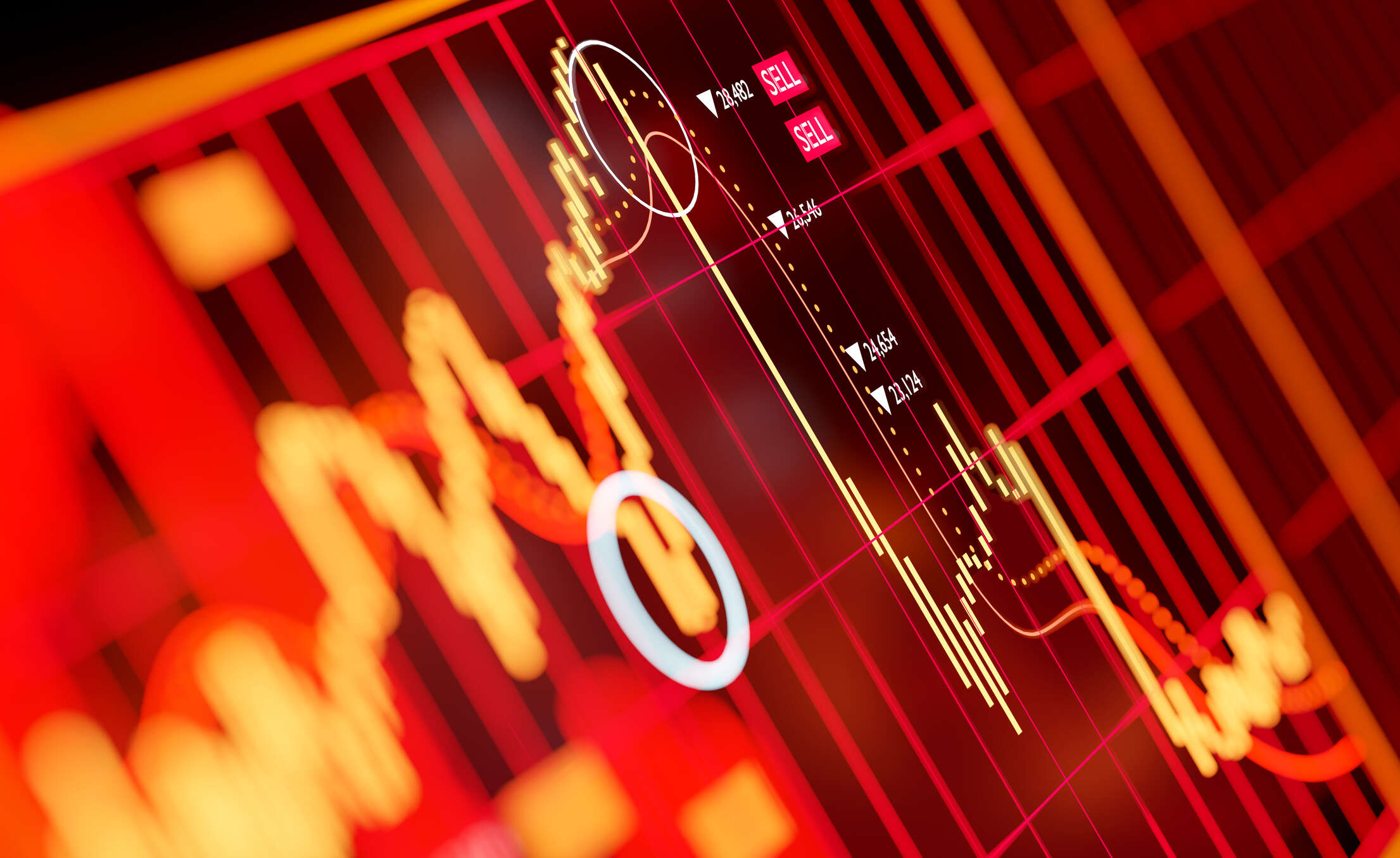
Artificial intelligence (AI) is increasingly used to scrutinise corporate behaviour, in particular by institutional investors seeking to understand the social impact of businesses in their portfolios. This, it is hoped, will bring added transparency to the world of corporate governance and provide incentives for companies to make meaningful improvements.
But it also presents risks. Companies may learn to game the algorithms that scrutinise their behaviour, for example, leading to a new form of ‘techno greenwashing’. And while AI-powered investment decisions could well provoke disputes and litigation, today’s legal frameworks are not clear on how these would be settled.

It is hoped that using AI to detect greenwashing will encourage companies to make more concrete commitments. (Photo by Solarseven/iStock)
Using AI to tackle greenwashing
“Greenwashing is a really dangerous thing,” says Fabiola Schneider, a doctoral student at University College Dublin. “It gives a false sense of security. If anyone can just say they’re [sustainable], why deploy resources if you don’t have to?”
Schneider is research co-lead for GreenWatch.AI, a project that uses natural language processing to hold companies to account for their sustainability statements, in particular their claims about decarbonisation. It does so by automatically identifying any claims a company makes about reducing carbon emissions and assessing their ‘boldness’. “We differentiate between different claims – there’s absolute claims, there are relative claims, you need to consider the time angle, and the angle of relativity. There’s a lot of nuance to it – and that’s where AI helps.”
The project started by analysing the claims companies made in the press and in their marketing messages, but it is now shifting its focus to public statements by senior executives to investors. “There’s a big difference in language between financial reports and, for example, advertisements and press releases that are designed for consumers. It’s very vague.”
GreenWatch.AI compares the boldness of these claims against a baseline 7% year-on-year reduction in emissions, the minimum required to limit global warming to 1.5C. If a company is making bold sustainability claims but only cutting emissions by this minimum or less, GreenWatch.AI’s model assigns it a high probability of being a greenwasher. “We never make an [absolute] assessment on whether someone is a greenwashing, we’re always giving a probability.”
Investors are just as prone to greenwashing as consumers.
Fabiola Schneider, GreenWatch.AI
One important audience for the tool will be institutional investors, such as banks, pension funds, and insurers, who are increasingly keen to ensure they are investing in sustainable businesses. “Investors are just as prone to greenwashing as consumers,” says Schneider. “When they build their portfolios, they don’t have the time to go through every report from the last three years and compare statements against actual numbers. They need someone to tell them, ‘Can I believe this information? Is this credible or not?'”
The hope is that by equipping investors with the ability to detect greenwashing, the tool will not only force companies to cut down on false claims but also to make more concrete commitments. Cracking down on greenwashing “will allow you to get credit if you actually increase your performance”, Schneider says. “We are hoping that this could even affect reporting by companies because they know if they exaggerate, someone is going to hold them accountable and it’s going to have a negative impact on their reputation and financial flows.”
AI and corporate governance: using machine learning to scrutinise companies
GreenWatch.AI is one of a growing number of initiatives that use AI to scrutinise the behaviour of companies and their senior leaders. Many of these have emerged in the environment, social and governance (ESG) investing space, where demand is high but information lacking.
Clarity.AI, for example, is a US start-up that aggregates diverse datasets to help investors to assess the social impact of the companies they invest in. The company uses machine learning to ‘triangulate’ data sets and identify correlations between variables. In January of this year, investment giant BlackRock acquired a minority stake in Clarity.AI and integrated its features into its Aladdin portfolio-management platform.
Other projects use AI to anticipate company performance. Evan Schnidman, an entrepreneur and data scientist, told Reuters last month that algorithmic analysis was able to detect doubt in the voices of IT industry CEOs as they downplayed the risk of the global chip shortage. This analysis would have predicted those companies’ eventual performance better than taking their words at face value, he said. Other investors are using AI to analyse the complexity of executive statements and indicators of company culture to gain fresh insights into financial performance.
It’s not just investors who are using AI to scrutinise businesses. Other examples include regulators – the US Securities and Exchange Commission uses AI to identify potential signs of insider trading; auditors – KPMG uses it to spot indicators of fraud in finance data; journalists – reporters at Ukraine’s Texty analysed satellite imagery to identify illegal mining; and activists – Amnesty International partnered with Element AI to document the scale of abuse that female politicians suffer on Twitter.
But for Florian Möslein, professor of law at the Philipps-University Marburg, the use of AI by institutional investors may be the most significant application in corporate governance. “It is probably even more important than the use of AI by companies and their boards,” he says, “because institutional investors are very much number crunchers, so they are basing their decisions on quantitative criteria. And that’s a field of application where AI is particularly useful.”
The risks and benefits of AI-powered scrutiny
The benefit of using AI to scrutinise corporate governance is its ability to draw on more data than would otherwise be possible, says Möslein. “The upside is that much more data can be digested, he says, “and it can be digested much more quickly.”
In the ESG space, any assistance in collecting and analysing data is welcome, says Charles Radclyffe, a partner at EthicsGrade, an ESG ratings agency focused on digital responsibility. “We’re definitely living in an ESG bubble,” he says, and companies are struggling with the volume of ESG-related data requests they receive from investors and analysts. “They’ve got an investor relations department of maybe five or ten people, in some cases, and they are absolutely swamped with requests for information. I think they’re very open to tools that make their lives easier, and the same is true on the investor side.”
Naturally, though, as in all areas of AI application, it is not without risks. Many tools and projects rely on natural-language processing. As investigated by Tech Monitor last week, an increasing concentration of NLP systems are built on a handful of ‘foundation models’, powerful algorithms trained on huge data sets. The growing pervasiveness of these models means that any flaws in their analysis could have far-reaching implications.
For Radclyffe, there is a danger that companies learn to ‘game’ the algorithms that are assessing their performance. “I think the risk is when a corporate might start to use machine learning to draft their disclosures in order to hit the highlights” – indicators that algorithms deem to be positive signals, he says. “And then you end up with a kind of a techno greenwashing, which will be even worse than we have right now.”
Möslein, meanwhile, says the opacity of many algorithms could lead to legal issues. “I think the most important risk we face here is transparency in decision making,” he says. “We never really know why decisions are taken by AI and there’s a risk of manipulation by the back door.”
We never really know why decisions are taken by AI and there’s a risk of manipulation by the back door.
Professor Florian Möslein, Philipps-University Marburg
This could well come to a head if an institutional investor is sued over a decision it made that was influenced by AI – something that Möslein believes will happen, sooner or later. “When it comes to litigation, you can always find out which human being has taken which decision. This human responsibility is more difficult to establish once you take advantage of AI.”
At the moment, however, it is unclear how disputes such as these would be handled. There is little overlap between regulation on corporate governance and on AI, Möslein explains. The EU’s forthcoming AI Act, for example, does not explain how, if at all, rules would be applied to AI-powered investment decisions.
“The risk classification that the AI Act is based on does not really fit well to the kind of decisions that we are talking about,” he explains. “They might be high-risk decisions for the person [making them] but they’re not high risk in the sense of the taxonomy of the AI Act.”
“My hope is that that there will be much more discussion on how corporate governance and securities regulation interact with technology-specific rules like the AI Act,” Möslein adds.