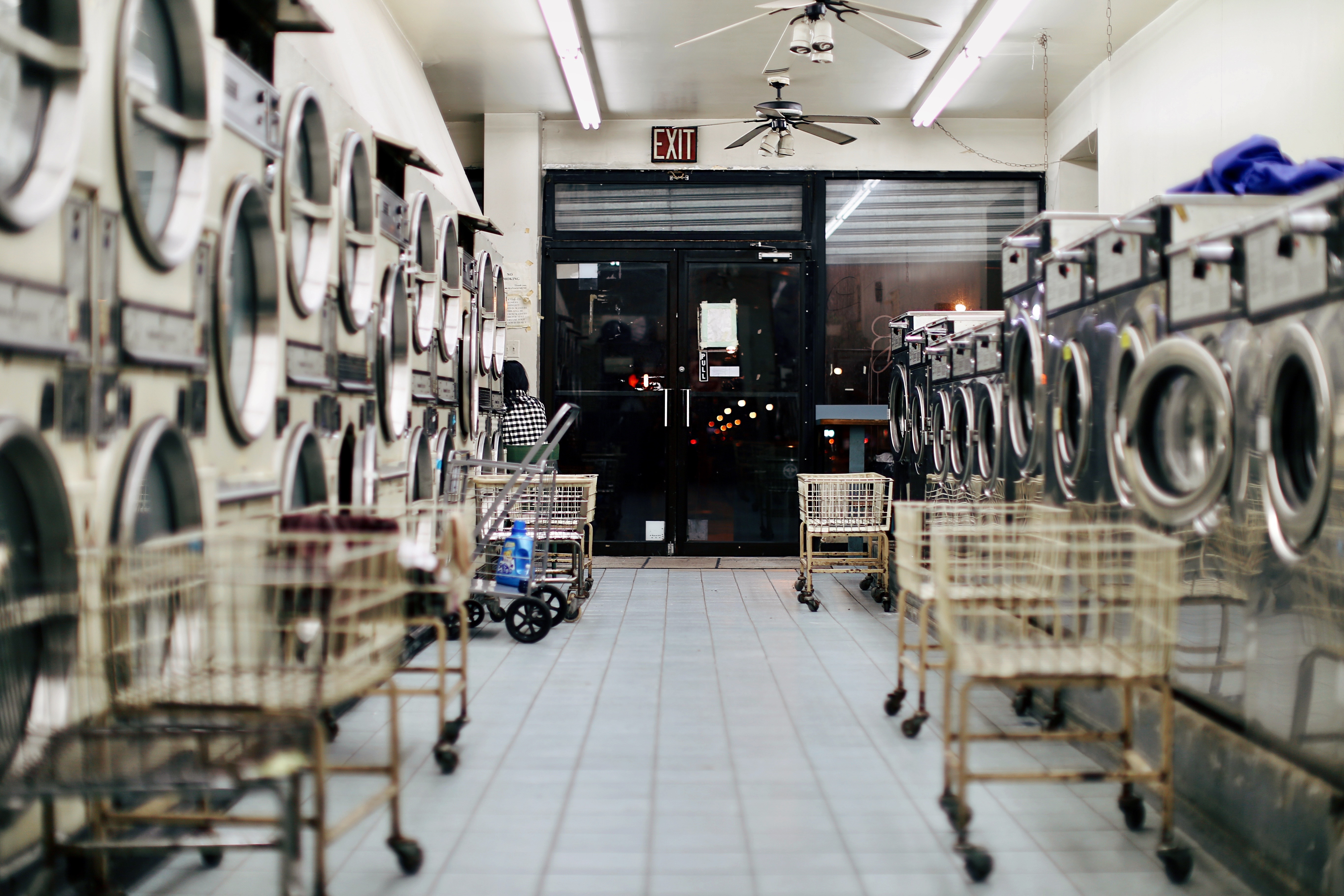
While 74% of business customers think banks use machine learning and artificial intelligence (AI) to spot money laundering, in reality most banks still rely almost solely on human investigators to manually sift through alerts, according to a new survey by global defence, aerospace and IT giant BAE Systems.
The lack of automation is having a major impact on efficiency and expense when it comes to the fight against money laundering, the company said. (Money laundering has been dubbed the “fifth largest economy in the world” and is estimated to cost the UK economy £24 billion a year).
Brian Ferro, Global Compliance Solutions Product Manager at BAE Systems Applied Intelligence, said: “Compliance investigators at banks can spend up to three days of their working week dealing with alerts – which most of the time are false positives.”
He added: “For banks to be on the front foot against money laundering, their investigators need to be supported by machine intelligence. Simplifying, optimising and automating the sorting of these alerts to give human investigators more time is the single most valuable thing banks and the compliance industry can do in the fight against money launderers. Right now, small improvements in efficiency of the systems banks use to find laundering can yield huge results.”
It’s a position echoed by Scott Zoldi, of analytic software firm FICO.
He told Computer Business Review: “There is a growing awareness that rules alone are often not sufficient to detect money laundering cases, and regulators have started pressuring the banks to adopt sophisticated analytics in their workflows.”
He added: “This is not surprising, as rules-based systems typically generate large amounts of false positives, resulting in thousands of false leads to be investigated. Further, rules reflect past expert knowledge but may not surface sophisticated new money laundering schemes designed to circumvent the rules in place.
Cryptocurrency fraud booms as hackers capitalise on hype
Robocop: How machine learning has its eyes set on internal expense fraud