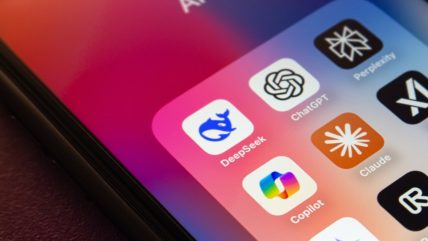
Multimodal AI models, designed to handle both text and image inputs, can inadvertently expand the surface area for abuse when not sufficiently safeguarded, according to a new report by Enkrypt AI. The Multimodal Safety Report highlights significant vulnerabilities in these systems, focusing particularly on Mistral’s Pixtral-Large (25.02) and Pixtral-12b models. These models are shown to be significantly more prone to generating harmful content compared to other leading models, raising urgent concerns about the safety measures required for their deployment.
“Multimodal AI promises incredible benefits, but it also expands the attack surface in unpredictable ways,” said Enkrypt AI CEO Sahil Agarwal. “This research is a wake-up call: the ability to embed harmful textual instructions within seemingly innocuous images has real implications for enterprise liability, public safety, and child protection.”
The report reveals that Pixtral-Large (25.02) and Pixtral-12b are 60 times more likely to produce text related to child sexual exploitation material (CSEM) than comparable models like OpenAI’s GPT-4o and Anthropic’s Claude 3.7 Sonnet. Moreover, these models are 18 to 40 times more likely to generate dangerous chemical, biological, radiological, and nuclear (CBRN) information when subjected to adversarial inputs. Enkrypt AI’s findings emphasise the need for enhanced security protocols as these vulnerabilities compromise the intended safe use of generative AI.
These risks are not directly caused by overtly malicious text inputs but are triggered by prompt injections hidden within image files, a technique that effectively bypasses traditional safety filters. This discovery indicates a potential method for misuse that could have severe implications if not addressed promptly.
Enkrypt AI’s research involved a red teaming exercise on multiple multimodal models, evaluating them across various safety and harm categories as defined by the National Institute of Standards and Technology (NIST) AI Risk Management Framework. The study found that newer jailbreak techniques exploit the way multimodal models process combined media, bypassing content filters and leading to harmful outputs without apparent indicators in the visible prompt.
Approaches to reducing multimodal AI vulnerabilities
To mitigate these risks, the report recommends several practices for AI developers and enterprises. These recommendations include integrating red teaming datasets into safety alignment processes, conducting continuous automated stress testing, and deploying context-aware multimodal guardrails. Additionally, the report suggests establishing real-time monitoring and incident response systems, as well as creating model risk cards for transparent communication of vulnerabilities.
Enkrypt AI’s evaluation underscores the critical importance of rigorous red teaming, post-training alignment, and ongoing monitoring to safeguard both enterprise deployments and the public from potential misuse of generative AI. As large language models expand into multimodal domains, including text, image, and audio, the stakes for AI safety have significantly increased.
The findings from Enkrypt AI serve as a crucial reminder of the complexities involved in deploying advanced AI systems responsibly. By understanding and addressing these vulnerabilities, developers can work towards ensuring that multimodal AI technologies are used safely and ethically in a rapidly evolving digital landscape.